GAN Inversion 整理
GAN Inversion 相关方法整理
《Image2StyleGAN: How to Embed Images Into the StyleGAN Latent Space?》
《Image2StyleGAN++: How to Edit the Embedded Images?》
《In-Domain GAN Inversion for Real Image Editing》
《Force-in-domain GAN inversion》
GAN Inversion 是指已知一张图和预训练好的 GAN 模型,找到使用 GAN 生成它的 Latent code,本文大部分文章使用的 GAN 是 StyleGAN 系列,找到图像在该模型上对应的 latent code。
现有 GAN inversion 方法主要存在两步:
- Encoder(Image to code):编码器难训练,表达容量有限。
- 优化编码器产生的 code
Image2StyleGAN: How to Embed Images Into the StyleGAN Latent Space?
通过像素级优化,找到输入图像对应的 latent code。
上图是使用 inversion latent code 生成的图进行人脸编辑的效果,最后一个眼镜加不上,说明网络找的 latent code 可能偏离了原始的 latent space。
本文探索了 StyleGAN 的嵌入算法和图像语义编辑的可能方案。
Image2StyleGAN++: How to Edit the Embedded Images?
本文与上一篇思路一样,依然是通过优化找到图的 latent code,上文只考虑了 w,本文在此基础上增加了 n。
本文改进了第一篇的算法,增加了细节提高了质量,本文方法还可以被开发很多应用。
In-Domain GAN Inversionfor Real Image Editing
网络结构
损失函数
本文加入鉴别器约束结果不要跳出语义空间。损失函数是来自图像空间而不是 latent code 空间,且在真实图像上进行训练。
通过微调编码器的 code 进行 domain-regularized 优化,损失函数见式 4,过程见图 2(b)。
Force-in-domain GAN inversion
网络结构
使用 Cycle loss 约束训练
本文提出了一种基于 in-domain GAN 的 force-in-domain GAN,它利用一个鉴别器来强制 inversion code 进入 latent space。force-in-domain GAN 也可以用稍加修改的 cycleGAN 来解释。
本文作者 : HeoLis
原文链接 : https://ishero.net/GAN%20Inversion%20%E6%95%B4%E7%90%86.html
版权声明 : 本博客所有文章除特别声明外,均采用 CC BY-NC-SA 4.0 许可协议。转载请注明出处!
学习、记录、分享、获得
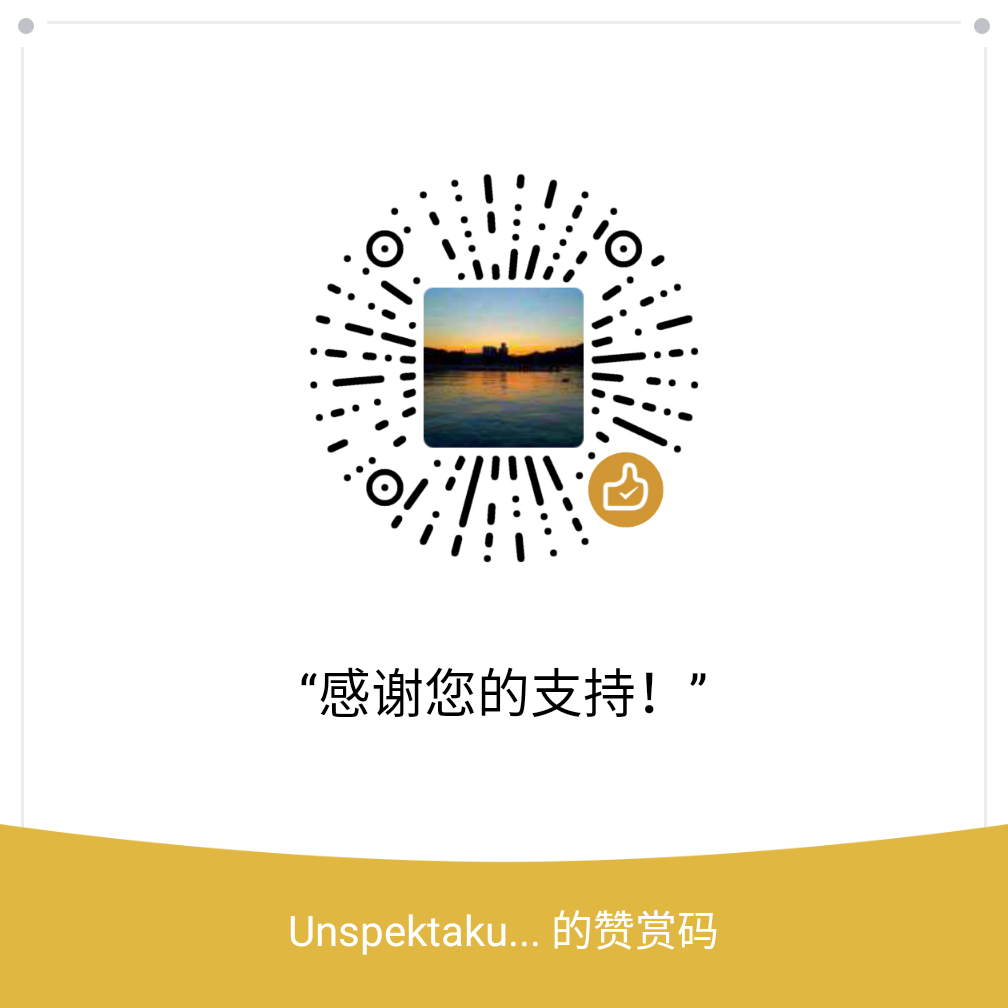